Table Of Content
- Statology Study
- Selecting Factors: Factor and Intervention Component Compatibility
- & 4. Measure Performance to Find an Effect
- 3. Factorial designs: Round 2¶
- 1.2. Measures of Different Constructs¶
- Minitab DOE Example
- Implementing Clinical Research Using Factorial Designs: A Primer
- Whether to Use an RCT or a Factorial Design
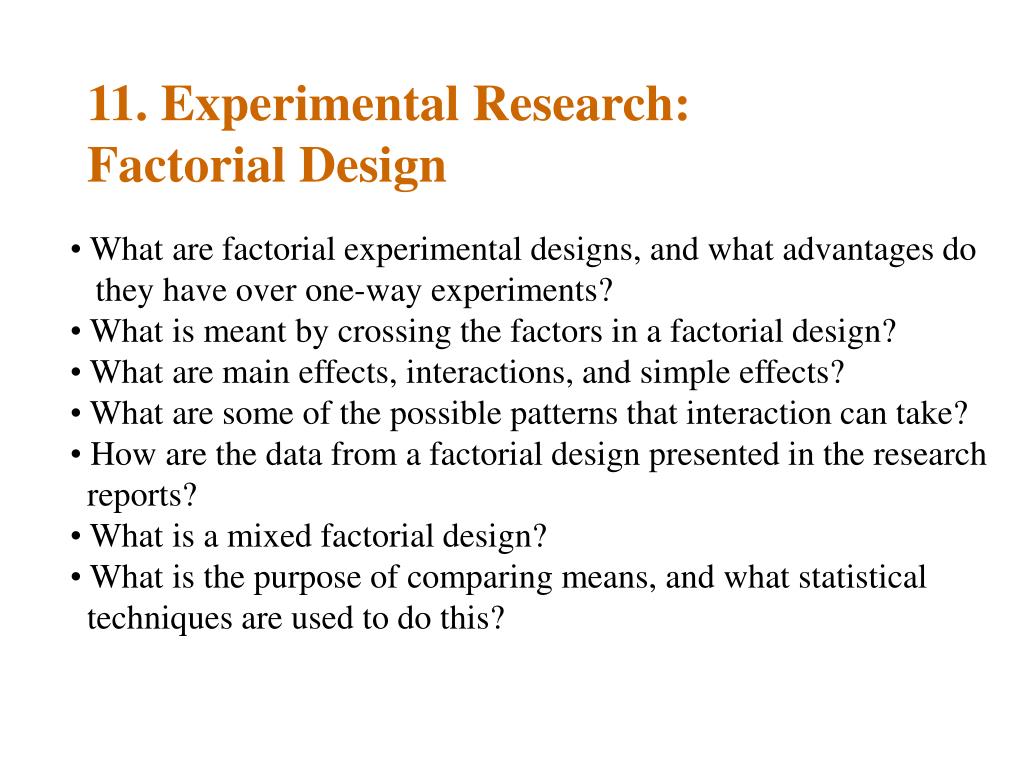
For example, imagine that a researcher wants to do an experiment looking at whether sleep deprivation hurts reaction times during a driving test. If she were only to perform the experiment using these variables–the sleep deprivation being the independent variable and the performance on the driving test being the dependent variable–it would be an example of a simple experiment. Let's look at the situation where we have one observation per cell. We need to think about where the variation occurs within this design. However, there are risks…if there is only one observation at each corner, there is a high chance of an unusual response observation spoiling the results. There would be no way to check if this was the case and thus it could distort the results fairly significantly.
Statology Study
These plots are different ways to present the statistical results of the analysis. Examples of these plots can be found in the Minitab Example for Centrifugal Contactor Analysis. The alpha value, which determines the limit of statistical significance, can be chosen in this menu also.
Selecting Factors: Factor and Intervention Component Compatibility
For all open access content, the Creative Commons licensing terms apply. Everyone gets the same pictures and does the same thing, so we're not actually testing anything. As expected, we see that the average height is 1 inch taller when subjects wear shoes vs. do not wear shoes.
& 4. Measure Performance to Find an Effect
Counselors could either reduce the intensity of an intervention component when it is one of many that a participant receives, or they could increase the intensity of an intervention component if the participant is receiving little other treatment. In short, maintaining treatment delivery fidelity may take more care, training and supervision in a factorial experiment than in an RCT. Including additional factors in an experiment might certainly affect the significance tests of a given factor. The reduction in power will be greater if we control experiment-wise error, due to the larger number of main and interaction effects. Including numerous factors might also increase the occurrence of interactions, which might affect the magnitude of a main effect (despite the lack of correlation between main and interaction effects with effect coding). Most complex correlational research, however, does not fit neatly into a factorial design.
3. Factorial designs: Round 2¶
In many factorial designs, one of the independent variables is a non-manipulated independent variable. One independent variable was disgust, which the researchers manipulated by testing participants in a clean room or a messy room. The other was private body consciousness, a variable which the researchers simply measured. Another example is a study by Halle Brown and colleagues in which participants were exposed to several words that they were later asked to recall [BKD+99]. Some were negative, health-related words (e.g., tumor, coronary), and others were not health related (e.g., election, geometry). The non-manipulated independent variable was whether participants were high or low in hypochondriasis (excessive concern with ordinary bodily symptoms).
In statistics, a full factorial experiment is an experiment whose design consists of two or more factors, each with discrete possible values or "levels", and whose experimental units take on all possible combinations of these levels across all such factors. A full factorial design may also be called a fully crossed design. Such an experiment allows the investigator to study the effect of each factor on the response variable, as well as the effects of interactions between factors on the response variable. One of the big advantages of factorial designs is that they allow researchers to look for interactions between independent variables. In this type of study, there are two factors (or independent variables), each with two levels.
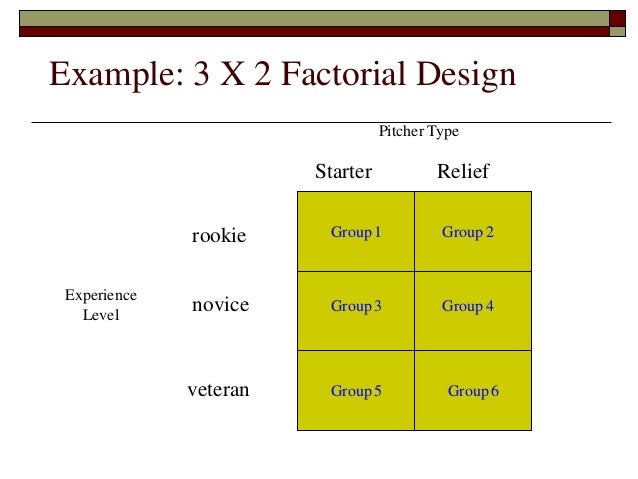
This would mean that each participant was tested in one and only one condition. In a within-subjects factorial design, all of the independent variables are manipulated within subjects. All participants could be tested both while using a cell phone and while not using a cell phone and both during the day and during the night. This would mean that each participant was tested in all conditions.
Implementing Clinical Research Using Factorial Designs: A Primer
But a multiple regression analysis including both income and happiness as independent variables would show whether each one makes a contribution to happiness when the other is taken into account. Research like this, by the way, has shown both income and health make extremely small contributions to happiness except in the case of severe poverty or illness [Die00]. There are many simple examples of two independent variables being dependent on one another to produce an outcome.
Whether to Use an RCT or a Factorial Design
Now we are going to shift gears and look at factorial design in a quantitative approach in order to determine how much influence the factors in an experiment have on the outcome. A null outcome situation is when the outcome of your experiment is the same regardless of how the levels within your experiment were combined. From the example above, a null outcome would exist if you received the same percentage of seizures occurring in patients with varying dose and age. The graphs below illustrate no change in the percentage of seizures for all factors, so you can conclude that the chance of suffering from a seizure is not affected by the dosage of the drug or the age of the patient.
We have plotted these effects against what we would expect if they were normally distributed. Let's look at another example in order to reinforce your understanding of the notation for these types of designs. Below is a figure of the factors and levels as well as the table representing this experimental space. These equations can be used as a predictive model to determine wt% methanol in biodiesel and number of theoretical stages achieved at different operating conditions without actually performing the experiments. However, the limits of the model should be tested before the model is used to predict responses at many different operating conditions.
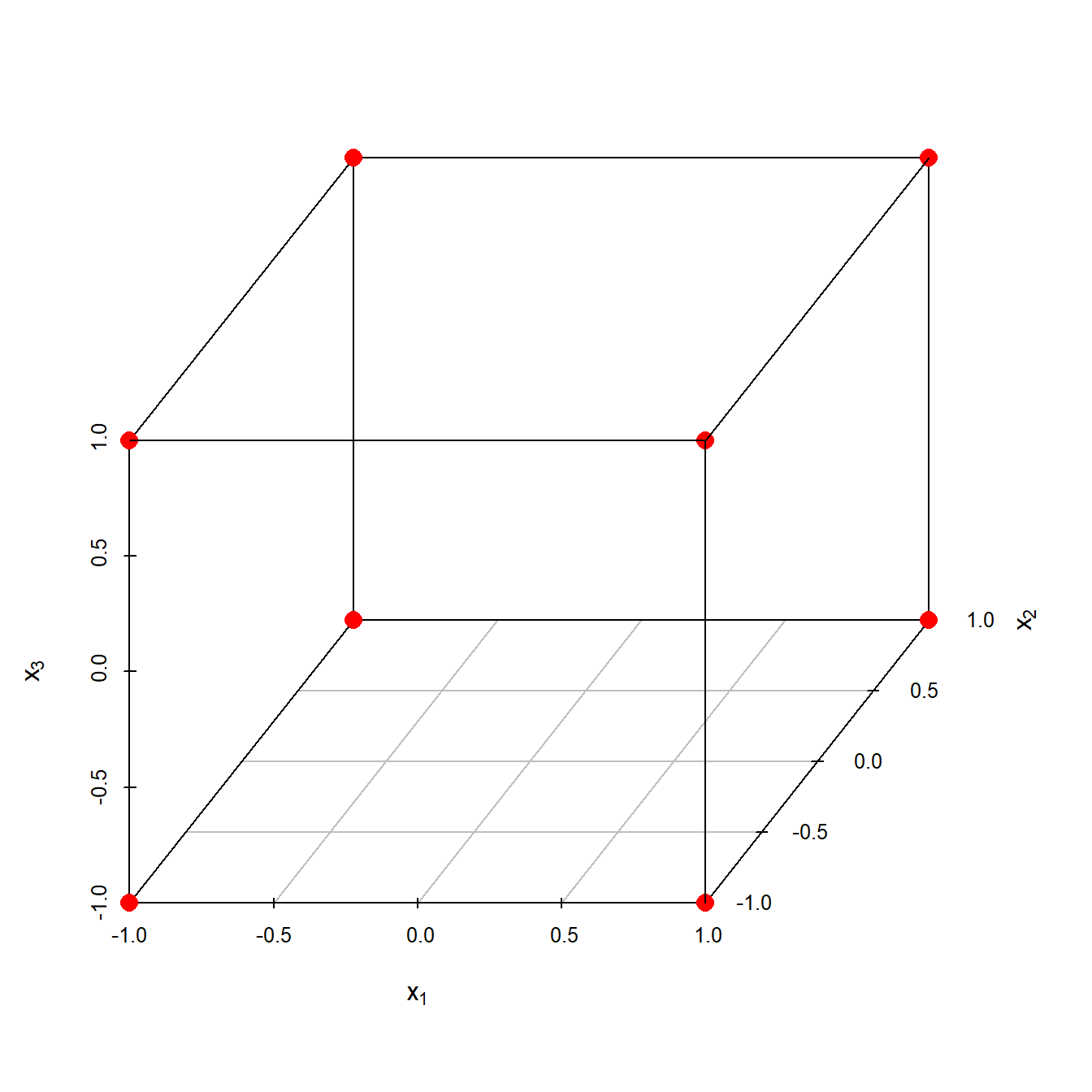
For example, Schnall and her colleagues were interested in how feeling disgusted affects the harshness of people’s moral judgments, but they were also curious about how disgust affects other variables, such as people’s willingness to eat in a restaurant. As another example, researcher Susan Knasko was interested in how different odors affect people’s behavior [Kna92]. She conducted an experiment in which the independent variable was whether participants were tested in a room with no odor or in one scented with lemon, lavender, or dimethyl sulfide (which has a cabbage-like smell). Although she was primarily interested in how the odors affected people’s creativity, she was also curious about how they affected people’s moods and perceived health—and it was a simple enough matter to measure these dependent variables too. Although she found that creativity was unaffected by the ambient odor, she found that people’s moods were lower in the dimethyl sulfide condition, and that their perceived health was greater in the lemon condition.
New Factor Ostro leaves old design in its wake - Cyclist
New Factor Ostro leaves old design in its wake.
Posted: Wed, 14 Feb 2024 08:00:00 GMT [source]
More specifically, in both cases, wearing a hat adds exactly 6 inches to the height, no more no less. When the factors are continuous, two-level factorial designs assume that the effects are linear. If a quadratic effect is expected for a factor, a more complicated experiment should be used, such as a central composite design. Optimization of factors that could have quadratic effects is the primary goal of response surface methodology. However, we can also perform a two-way ANOVA to formally test whether or not the independent variables have a statistically significant relationship with the dependent variable.
It is possible to create factorial, response surface, mixture, and taguchi method DOEs in Minitab. The general method for creating factorial DOEs is discussed below. The examples discussed in this section only scratch the surface of how researchers use complex correlational research to explore possible causal relationships among variables.
But in many ways, the complex design of this experiment undertaken by Schnall and her colleagues is more typical of research in psychology. Fortunately, we have already covered the basic elements of such designs in previous chapters. In this chapter, we look closely at how and why researchers combine these basic elements into more complex designs. We start with complex experiments—considering first the inclusion of multiple dependent variables and then the inclusion of multiple independent variables. For the vast majority of factorial experiments, each factor has only two levels. For example, with two factors each taking two levels, a factorial experiment would have four treatment combinations in total, and is usually called a 2×2 factorial design.
No comments:
Post a Comment